AutoBasisEncoder: Pre-trained Neural Field Basis via Autoencoding for Operator Learning
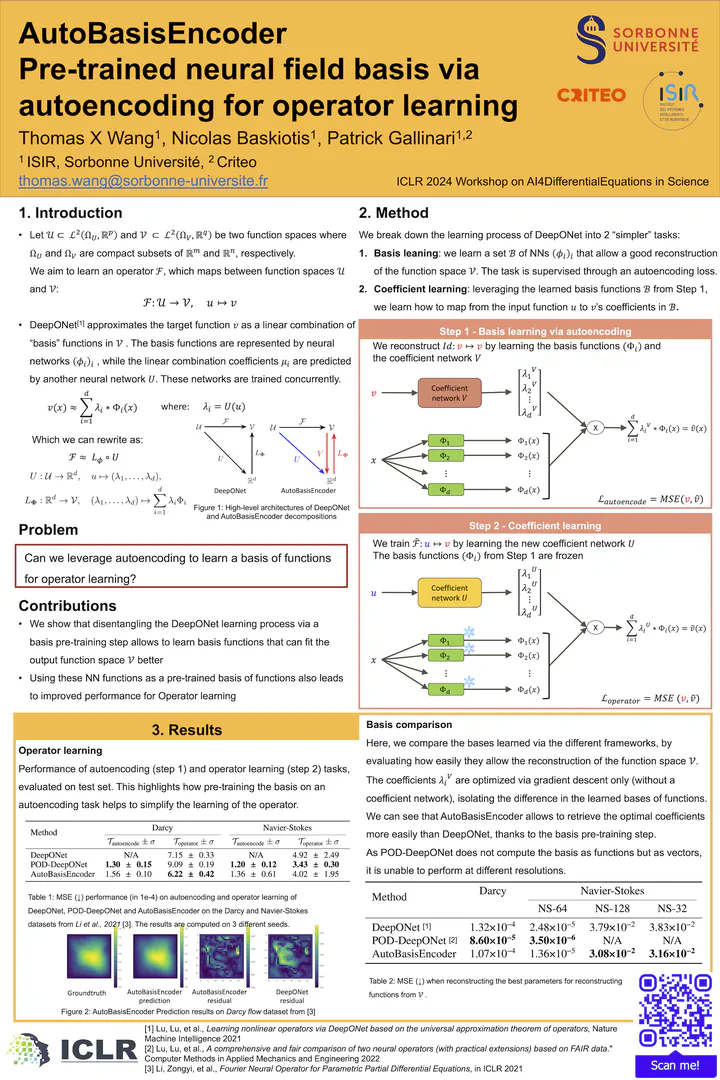
Abstract
We introduce AutoBasisEncoder, a novel framework designed for operator learn- ing – the task of learning to map from one function to another. This approach au- tonomously discovers a basis of functions optimized for the target function space and utilizes this pre-trained basis for efficient operator learning. By introducing an intermediary auto-encoding task to the popular DeepONet framework, AutoBa- sisEncoder disentangles the learning of the basis functions and of the coefficients, simplifying the operator learning process. Initially, the framework learns basis functions through auto-encoding, followed by leveraging this basis to predict the coefficients of the target function. Preliminary experiments indicate that Auto- BasisEncoder’s basis functions exhibit superior suitability for operator learning and function reconstruction compared to DeepONet. These findings underscore the potential of AutoBasisEncoder to enhance the landscape of operator learning frameworks
Type
Publication
In ICLR 2024 Workshop on AI4DifferentialEquations In Science